In recent years, neuromorphic computing has emerged as a groundbreaking approach to creating more efficient and brain-like artificial intelligence (AI) systems. Rooted in mimicking the architecture and functionality of the human brain, this technology holds the potential to revolutionize not only AI but also cognitive systems, robotics, and beyond. North America, particularly the U.S. and Canada, is at the forefront of neuromorphic computing innovation, with academic institutions, tech companies, and research organizations leading the charge in its development. As the demand for more adaptive, efficient, and intelligent AI systems grows, neuromorphic computing stands poised to reshape industries ranging from healthcare and robotics to automotive and beyond.
Neuromorphic computing is a field of computing that aims to design systems based on the structure and function of the brain’s neural networks. Traditional computing models, based on binary logic and sequential processing, differ significantly from the parallel processing and dynamic adaptability seen in biological neural networks. Neuromorphic systems use specialized hardware (neuromorphic chips) and algorithms that mimic how neurons and synapses communicate and adapt in the brain. These systems are highly efficient, capable of processing massive amounts of data with minimal power consumption, and can perform tasks like pattern recognition, decision-making, and learning in real-time.
Unlike conventional computers, which require large amounts of energy to perform AI tasks, neuromorphic chips are designed to operate with power efficiency comparable to the human brain. This capability has made them particularly attractive for edge AI applications, where processing power needs to be distributed close to the data source, such as in autonomous vehicles, robotics, and IoT devices.
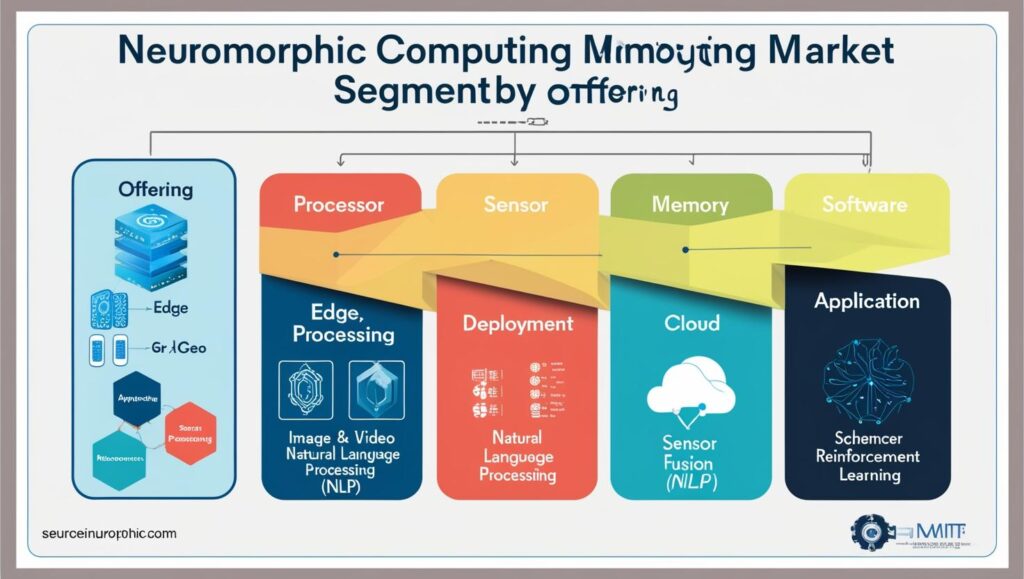
The Growing Role of Neuromorphic Computing in North America
In North America, neuromorphic computing is seen as one of the key technologies to accelerate the development of next-gen AI systems. As companies strive for more intelligent, energy-efficient systems, the region’s research community and tech industry are rapidly embracing this new paradigm. Below, we explore the key factors driving the growth and adoption of neuromorphic computing in North America.
1. The Demand for More Powerful and Efficient AI Systems
The advent of AI and machine learning has led to an increasing demand for systems that can process vast amounts of data in real-time. Conventional AI processors, such as GPUs, have driven innovation in many AI applications. However, these processors still consume significant amounts of energy and often struggle with tasks requiring adaptive learning and real-time processing. Neuromorphic computing offers a solution to these limitations by providing a more efficient way to simulate cognitive functions and handle complex data sets with much lower energy consumption.
As AI applications become more integrated into daily life — from autonomous vehicles to smart cities — the need for more scalable and energy-efficient AI models will only increase. Neuromorphic chips are uniquely suited to handle the computational load of these applications while providing faster, more accurate responses to dynamic environments.
2. North American Investment in Neuromorphic Research and Development
North America has long been a hub for technological innovation, and the field of neuromorphic computing is no exception. Leading universities, national laboratories, and tech companies in the U.S. and Canada are investing heavily in neuromorphic research and development. For example, IBM, one of the pioneers of neuromorphic computing, developed the TrueNorth chip, which simulates a brain-like network of neurons to power AI applications. Similarly, Intel has made significant strides in the field with its Loihi neuromorphic processor, designed for AI and machine learning tasks.
Research institutions such as Stanford University, MIT, and the University of Toronto are also conducting cutting-edge research in neuromorphic computing, exploring novel architectures, learning algorithms, and hardware innovations. This investment is creating a fertile ecosystem for the development of neuromorphic technologies and is expected to drive further innovation.
Download PDF Brochure @
https://www.marketsandmarkets.com/pdfdownloadNew.asp?id=227703024
3. Enabling the Future of Autonomous Systems and Robotics
The rise of autonomous systems and robotics presents one of the most promising applications for neuromorphic computing. These systems, including self-driving cars, autonomous drones, and industrial robots, require the ability to process information from multiple sensors and make decisions in real-time, often with limited computational resources. Neuromorphic computing, with its low-power consumption and ability to process sensory data in parallel, is an ideal solution for these applications.
Neuromorphic chips are particularly well-suited for edge AI systems, where processing needs to occur close to the source of data. For instance, a self-driving car needs to process data from its cameras, LiDAR, and radar sensors in real-time to navigate complex environments. By incorporating neuromorphic processors, autonomous vehicles can perform these tasks more efficiently, with less reliance on cloud computing, reducing latency and improving overall system performance.
4. Impact on Healthcare and Biomedical Applications
Neuromorphic computing is also poised to have a profound impact on healthcare and biomedical applications. With the increasing complexity of medical data, such as genomics, medical imaging, and electrocardiograms (ECGs), healthcare professionals need AI systems that can rapidly process large datasets while making real-time decisions. Neuromorphic computing offers the ability to model complex biological systems and simulate the behavior of neurons and synapses, making it a powerful tool for healthcare innovation.
For example, neuromorphic systems could be used for predictive analytics, identifying patterns in patient data to detect early signs of diseases like cancer or neurological disorders. Additionally, they could help design advanced prosthetics or assistive technologies by providing more adaptive, human-like control systems for devices such as exoskeletons or robotic limbs.
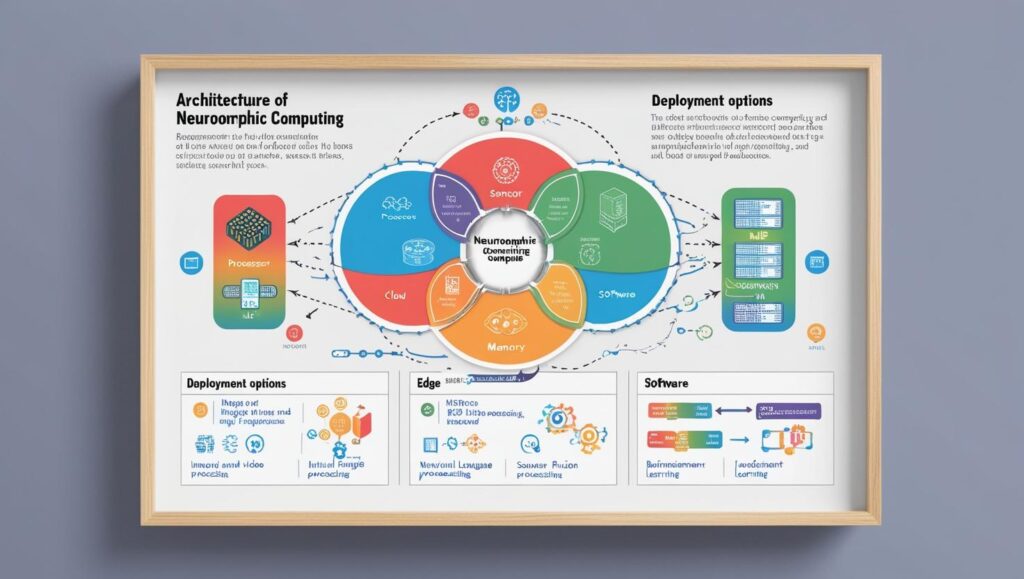
Challenges and Opportunities in Neuromorphic Computing
While the potential for neuromorphic computing is immense, the industry still faces several challenges, including:
Hardware limitations: Although neuromorphic chips are highly efficient, they are still in the early stages of development, and scaling them for widespread use will require significant advancements in chip design and production capabilities.
Algorithmic challenges: Neuromorphic systems need specialized learning algorithms to function effectively. Developing these algorithms is an ongoing research effort, as the field of neuromorphic computing is still evolving.
Cost and accessibility: Neuromorphic chips are currently expensive and relatively inaccessible to smaller organizations. However, as the technology matures and demand increases, costs are expected to come down, making it more accessible to a broader range of industries.
Despite these challenges, the opportunities in neuromorphic computing are vast. As industries look to implement more adaptive AI, energy-efficient computing, and real-time decision-making systems, neuromorphic computing will continue to play a pivotal role.
The Road Ahead: The Future of Neuromorphic Computing in North America
The future of neuromorphic computing in North America looks incredibly promising. With continued investment in research and development, growing industry adoption, and the demand for more efficient AI systems, neuromorphic computing is set to redefine the capabilities of modern computing. From autonomous vehicles and smart cities to healthcare and biometrics, this technology promises to unlock new frontiers in AI, making it a core pillar of future technological advancements.
As North America continues to lead the charge in neuromorphic innovation, we are likely to see major breakthroughs in both the hardware and software that will enable smarter, more human-like machines capable of adapting to an increasingly complex world.