Neuromorphic computing, a paradigm inspired by the structure and functioning of the human brain, is on the verge of transforming the artificial intelligence (AI) landscape. Unlike traditional computing systems, which rely on binary logic and sequential processing, neuromorphic computing leverages spiking neural networks (SNNs) to process information in a highly parallel, event-driven manner. This makes it more efficient, adaptive, and scalable, setting the stage for unprecedented advances in AI, robotics, autonomous systems, and beyond.
As the demand for smarter, energy-efficient systems grows, the neuromorphic computing industry is gaining momentum. With its ability to mimic brain-like processes, it is poised to revolutionize how machines learn, make decisions, and interact with the world. This article explores the future of the neuromorphic computing industry, focusing on emerging trends, technological breakthroughs, and the potential impact on various sectors.
The neuromorphic computing market size is expected to grow from USD 28.5 million in 2024 and is estimated to reach USD 1,325.2 million by 2030; it is expected to grow at a Compound Annual Growth Rate (CAGR) of 89.7% from 2024 to 2030. Growth in the neuromorphic computing market is driven through the integration of neuromorphic computing in automotive and space operations. In space, where bandwidth is limited and the communication delay might be considered large, onboard processing capabilities are crucial. The neuromorphic processor analyzes and filters data at the point of collection, reducing the need to transmit large datasets back to Earth. whereas, in automobile sector, neuromorphic processors can make autonomous driving systems more responsive by onboard real-time processing with minimal latency so that safety is ensured along with efficiency.
Future of Neuromorphic Computing Industry include
1. Neuromorphic Computing: The Brain-Inspired Revolution
The core idea behind neuromorphic computing is to replicate the brain’s ability to process and store information. While traditional processors handle tasks sequentially, neuromorphic systems process data in parallel, simulating the way neurons and synapses communicate in the human brain. This brain-inspired approach enables event-driven processing, meaning that computations are only performed when necessary, significantly reducing energy consumption compared to traditional computing models.
This fundamental shift in computing architecture allows neuromorphic systems to handle complex tasks like pattern recognition, real-time decision-making, and adaptive learning with much greater efficiency. As neuromorphic chips become more sophisticated, they will increasingly drive advancements in areas that require rapid, low-power processing, such as autonomous vehicles, smart cities, edge computing, and robotics.
2. Industry Growth and Market Trends
The neuromorphic computing market is poised for significant growth in the coming years. According to recent market reports, the global neuromorphic computing market is expected to expand at a CAGR of over 89.7% through the next decade. This growth will be fueled by demand across several industries for more energy-efficient and intelligent systems.
Key trends driving the industry forward include:
Increased Demand for AI-Powered Devices: As AI becomes more integrated into everyday applications, from consumer electronics to industrial systems, the need for faster, more efficient processing will drive adoption of neuromorphic systems. This includes everything from smartphones and wearables to autonomous vehicles and drones.
Advancements in Chip Design: Companies like Intel with its Loihi chip and IBM with its TrueNorth are leading the charge in developing neuromorphic processors. These chips are designed to mimic the brain’s neural architecture, enabling lower power consumption and greater processing capacity for tasks such as image recognition, speech processing, and sensor fusion.
Integration with Edge Computing: Neuromorphic computing’s efficiency and low latency make it ideal for edge computing applications, where devices need to process data locally without relying on cloud-based systems. In industries like healthcare, manufacturing, and IoT, this enables real-time analytics and decision-making at the edge.
AI and Machine Learning Advancements: The ability of neuromorphic systems to support unsupervised learning and adapt in real-time gives them an edge over traditional systems, especially for applications that require dynamic learning from unstructured data. This is particularly relevant for robotics, where neuromorphic chips can enable robots to learn new tasks without extensive retraining.
3. Key Applications Transforming Industries
The neuromorphic computing industry is set to disrupt a wide range of sectors by providing solutions that were once considered impractical with conventional computing models. Here are some key applications where neuromorphic systems are already making an impact—and where they will continue to push the boundaries of possibility.
Autonomous Systems and Robotics
In autonomous systems, the ability to process and react to sensory information in real-time is critical. Neuromorphic chips are designed to handle these tasks more efficiently than traditional computing systems by mimicking the brain’s sensory processing capabilities. For instance, autonomous vehicles rely on sensors like LiDAR, cameras, and radar to understand their environment and make decisions. Neuromorphic systems, with their ability to process massive amounts of sensory data in parallel, can significantly improve the speed and accuracy of decision-making, making autonomous systems safer and more reliable.
Additionally, in the robotics industry, neuromorphic computing can help robots navigate complex environments, interact with humans, and learn new tasks more effectively. The ability of neuromorphic systems to mimic the brain’s adaptability means that robots can continue to learn and improve their performance over time, making them more autonomous and versatile in various applications, from manufacturing to healthcare.
Download PDF Brochure @
https://www.marketsandmarkets.com/pdfdownloadNew.asp?id=227703024
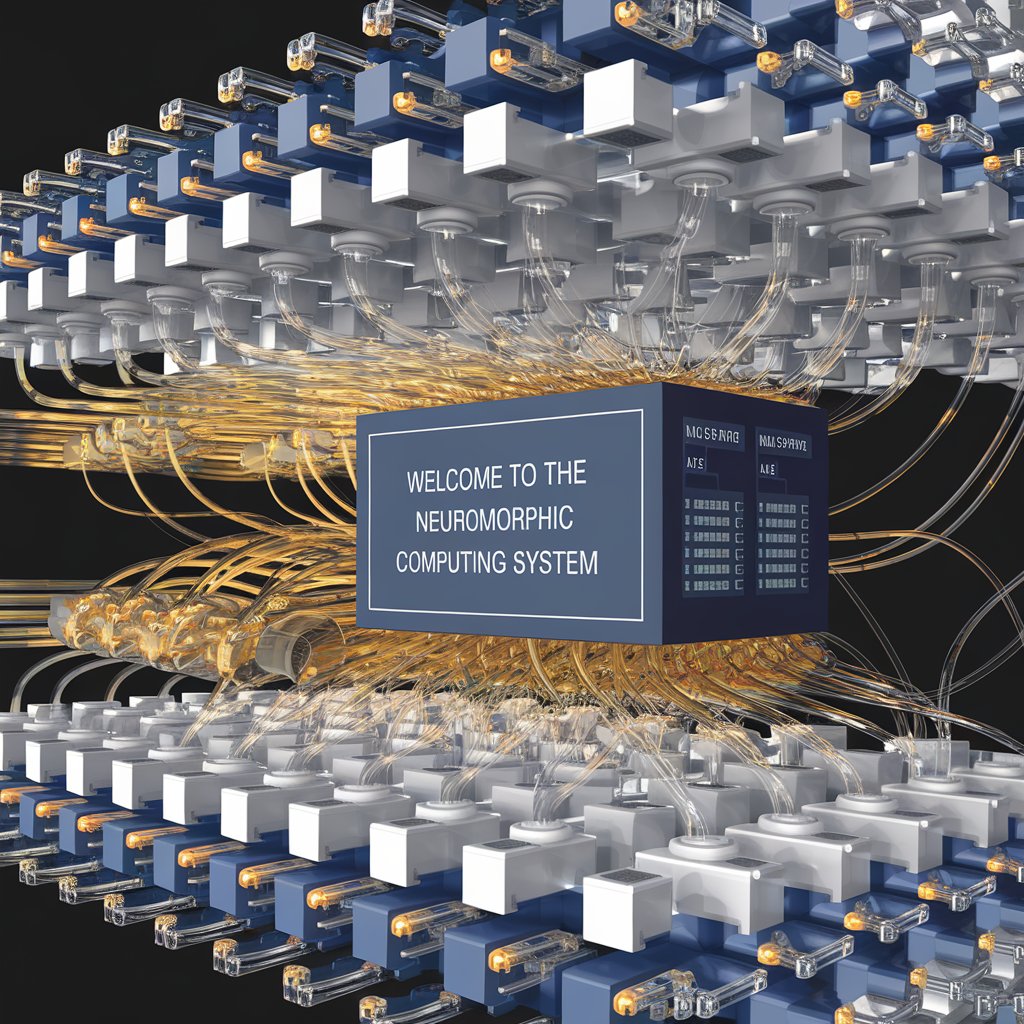
Healthcare and Medical Devices
In healthcare, neuromorphic computing has the potential to improve diagnostic tools, personalized medicine, and assistive devices. For instance, in medical imaging, neuromorphic systems can process data from MRI scans or X-rays faster and more accurately, identifying patterns or anomalies that might be missed by human doctors or conventional AI models.
In wearable devices for health monitoring, neuromorphic computing allows for the continuous, real-time processing of data, enabling proactive health management. These systems can analyze biometric data—such as heart rate, blood pressure, and blood oxygen levels—and alert users to potential health risks before they become critical, all while consuming minimal power.
Smart Cities and Edge Computing
As urban areas become smarter and more connected, neuromorphic computing will play a pivotal role in processing data generated by various IoT devices deployed throughout a city. From traffic management to public safety, neuromorphic systems can quickly process real-time sensor data and make decisions that improve efficiency and safety.
For example, in traffic control, neuromorphic systems can analyze real-time data from cameras and sensors to optimize traffic flow, reduce congestion, and improve emergency response times. Similarly, smart surveillance systems can process video feeds to detect unusual activity or identify potential threats, providing enhanced security without relying on cloud-based processing, which can introduce delays.
Cybersecurity and Data Privacy
Neuromorphic systems also offer promising applications in cybersecurity. Their ability to recognize patterns and anomalies quickly can help detect cybersecurity threats in real time, preventing data breaches and mitigating risks before they escalate. Neuromorphic computing’s fault tolerance and distributed nature make these systems highly resistant to attacks, adding an extra layer of protection for critical infrastructure.
4. Challenges and the Road Ahead
Despite the immense potential, the neuromorphic computing industry still faces several challenges. The design and development of neuromorphic chips are complex, requiring new algorithms, architectures, and hardware that differ from traditional computing systems. Moreover, the lack of standardization across neuromorphic systems may hinder large-scale adoption.
Additionally, scaling up neuromorphic systems to meet the demands of various industries requires further breakthroughs in both hardware and software. Researchers are focused on improving the efficiency of neuromorphic chips, increasing their processing power, and ensuring that they can be integrated seamlessly into existing systems.
However, as major tech companies and research institutions continue to push the envelope in neuromorphic computing, we can expect these challenges to be overcome. Continued investment in AI research, brain-inspired architectures, and neuromorphic software development will likely accelerate the growth of the industry.
5.A Transformative Future
The future of neuromorphic computing is incredibly promising, with the potential to reshape industries, revolutionize AI, and enable smarter, more efficient systems. From autonomous vehicles to healthcare and edge computing, neuromorphic systems will drive innovations that were once considered science fiction.
As the technology matures and adoption increases, we can expect to see more widespread integration of neuromorphic chips into everyday devices, from smartphones to drones, enabling a new era of intelligent systems capable of real-time learning, adaptive decision-making, and energy-efficient processing.
With the global neuromorphic computing market set to experience exponential growth, the next few years will be critical in determining how quickly and effectively this transformative technology is adopted across industries. As we move closer to achieving brain-like AI capabilities, the neuromorphic computing industry will undoubtedly be at the forefront of the next great technological revolution.
Key companies operating in the neuromorphic computing companies are Intel Corporation (US), IBM (US), Qualcomm Technologies, Inc. (US), Samsung Electronics Co., Ltd. (South Korea), Sony Corporation (Japan), BrainChip, Inc. (Australia), SynSense (China), MediaTek Inc. (Taiwan), NXP Semiconductors (Netherlands), Advanced Micro Devices, Inc. (US), Hewlett Packard Enterprise Development LP (US), OMNIVISION (US), among others.
Frequently Asked Questions
1. What is Neuromorphic Computing?
Neuromorphic computing is an innovative computing paradigm inspired by the structure and functioning of the human brain. It uses spiking neural networks (SNNs) to process information in a way that mimics the brain’s neural architecture, enabling energy-efficient, parallel, and adaptive processing of data. Neuromorphic systems are designed to simulate the behavior of neurons and synapses, offering a more biologically plausible approach to computing.
2. How does Neuromorphic Computing differ from traditional computing?
Traditional computing relies on sequential processing using binary logic (1s and 0s), while neuromorphic computing uses parallel, event-driven processing, similar to the way neurons in the human brain work. This enables real-time data processing, reduced power consumption, and the ability to learn from data more effectively. Unlike traditional systems, neuromorphic computing doesn’t require a central clock, allowing for greater efficiency in handling complex tasks such as machine learning, pattern recognition, and autonomous decision-making.
3. What are the main advantages of Neuromorphic Computing?
Key advantages of neuromorphic computing include:
- Energy efficiency: Neuromorphic systems are highly power-efficient compared to traditional computing, making them ideal for battery-powered and mobile devices.
- Real-time processing: These systems can process data and make decisions in real-time, without the delays associated with traditional cloud-based or sequential systems.
- Adaptability: Neuromorphic systems can learn from data autonomously and adjust their behavior over time, much like the human brain.
- Fault tolerance: Neuromorphic systems are more resilient to hardware failures due to their distributed nature, allowing them to continue functioning even when individual components fail.
- Scalability: Neuromorphic systems can scale efficiently, handling increasing amounts of data without losing performance.
4. What industries will benefit the most from Neuromorphic Computing?
Neuromorphic computing will benefit several industries, including:
- Autonomous vehicles: Enhances real-time decision-making and sensory processing for safer, more efficient driving systems.
- Healthcare: Enables faster, more accurate medical diagnostics, real-time patient monitoring, and personalized medicine.
- Robotics: Powers intelligent, adaptive robots capable of learning from their environment and performing complex tasks.
- Smart cities and IoT: Improves real-time data processing and decision-making for applications like traffic management, energy optimization, and security systems.
- Cybersecurity: Detects anomalies and cyber threats in real-time, enhancing protection against data breaches and attacks.
5. What challenges does Neuromorphic Computing face?
Despite its potential, neuromorphic computing faces several challenges:
- Complex chip design: Neuromorphic hardware and software are still in the developmental stages, and designing effective neuromorphic chips that can scale is a complex task.
- Standardization: There is currently a lack of industry-wide standards, making it difficult to integrate neuromorphic systems across various applications and devices.
- Limited adoption: The adoption of neuromorphic computing is still relatively niche, with mainstream industries gradually integrating the technology as it matures.
- Software and algorithm development: Building software and algorithms that can fully leverage neuromorphic hardware remains an ongoing challenge.
6. What are some real-world examples of Neuromorphic Computing in use?
Some notable real-world applications of neuromorphic computing include:
- Intel’s Loihi chip: A neuromorphic chip that mimics the brain’s processing abilities and is used for real-time learning, sensory processing, and robotics applications.
- IBM’s TrueNorth chip: A brain-inspired chip that has been used for research in AI, machine learning, and robotics, simulating large-scale neural networks with low power consumption.
- Neuromorphic robots: Robots that use neuromorphic systems to adapt to their environment, learn new tasks, and process sensory data in real-time for more intelligent and autonomous behavior.