The Edge AI hardware market is quickly evolving, with technologies like artificial intelligence (AI) and machine learning (ML) becoming increasingly integrated into everyday devices. By enabling data processing at the edge of networks—close to where data is generated—Edge AI hardware provides numerous advantages, including faster decision-making, reduced latency, and enhanced privacy. However, despite its rapid growth and potential, there are several opportunities and challenges ahead for this emerging market.
Opportunities in the Edge AI Hardware Market
- Expansion Across Industries Edge AI hardware is making significant inroads across multiple industries, including automotive, healthcare, manufacturing, and smart cities. In autonomous vehicles, for example, real-time data processing via Edge AI hardware allows systems to make split-second decisions, such as adjusting speed or avoiding obstacles. Similarly, healthcare applications benefit from Edge AI’s ability to analyze patient data locally, leading to more immediate and accurate diagnoses, thus reducing the reliance on centralized cloud systems.
The Internet of Things (IoT) is also a major driver, with billions of connected devices generating continuous streams of data. Edge AI technology allows these devices to make intelligent decisions without the need for constant communication with centralized cloud servers, thus optimizing operations and cutting down on bandwidth usage. As IoT adoption continues to expand, Edge AI hardware will play a pivotal role in enabling the next wave of connected, intelligent devices.
- Improved Data Privacy and Security One of the most compelling advantages of Edge AI hardware is its ability to enhance data privacy and security. By processing data locally, Edge AI minimizes the amount of sensitive information that needs to be transmitted across networks, reducing the risk of cyberattacks and data breaches. This is particularly crucial in sectors like finance and healthcare, where regulatory requirements demand strict control over data handling and privacy.
Moreover, local data processing means that businesses can comply with data sovereignty laws and regulations that restrict the storage and movement of data across national borders. As concerns about privacy grow globally, more businesses will likely turn to Edge AI hardware as a secure solution.
- Cost-Efficiency and Energy Savings Traditional cloud computing often requires substantial infrastructure investments and energy consumption. Edge AI hardware, however, offers a more energy-efficient alternative. By processing data on the edge of the network rather than in a centralized cloud, organizations can reduce the energy required for data transmission and server-side computations. This results in significant cost savings, especially for businesses operating in energy-constrained environments such as remote locations or industrial IoT systems.
Download PDF Brochure @ https://www.marketsandmarkets.com/pdfdownloadNew.asp?id=158498281
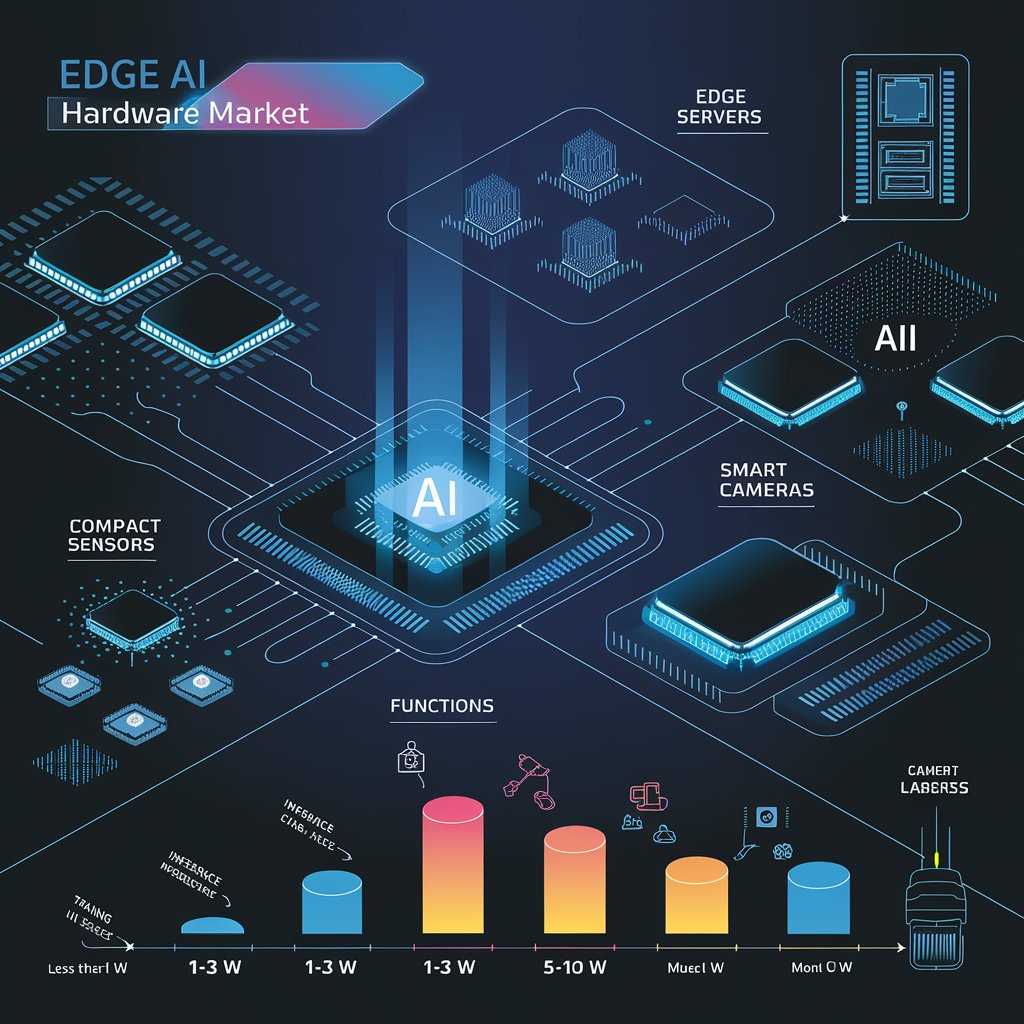
Challenges in the Edge AI Hardware Market
- High Initial Investment One of the primary barriers to widespread adoption of Edge AI hardware is the high initial cost of implementation. Developing and deploying edge devices with built-in AI capabilities requires significant investment in specialized hardware, including processors and sensors capable of handling AI workloads. Additionally, businesses need to invest in infrastructure to support these devices, such as local storage solutions and secure communication networks.
While the long-term benefits, such as reduced operational costs and improved efficiency, are evident, many small and medium-sized enterprises (SMEs) may struggle with the initial financial burden of Edge AI hardware adoption.
- Integration Complexity Another challenge lies in the integration of Edge AI hardware with existing systems. Many businesses have established IT and network infrastructures, and incorporating Edge AI devices into these systems may require significant updates or modifications. Seamless interoperability between Edge AI hardware, traditional IT infrastructure, and cloud systems is crucial to ensuring the success of an Edge AI deployment. Without robust integration, businesses risk inefficiencies, data silos, and compromised performance.
Additionally, managing and maintaining a distributed network of Edge AI devices can be more complex than maintaining centralized systems. Organizations must develop new skill sets and ensure proper training for their workforce to maximize the effectiveness of these devices.
- Scalability Issues While Edge AI hardware is ideal for localized, real-time decision-making, scaling these solutions across larger networks presents a challenge. As businesses increase their deployments of Edge AI devices, they need to ensure that the infrastructure can scale efficiently without creating bottlenecks or overwhelming local processing capabilities. Edge AI solutions must be able to handle vast amounts of real-time data from multiple sources while maintaining low latency and high processing power. The complexity of managing multiple edge nodes in diverse environments becomes exponentially greater as the number of devices and the volume of data increases.
- Limited Processing Power Edge devices typically have more limited processing power compared to centralized cloud-based servers. While this is improving with advancements in hardware, there are still certain AI models and workloads that require more computational resources than edge devices can provide. This limitation may hinder the deployment of more advanced AI applications at the edge, particularly in areas such as deep learning, which requires heavy computing capabilities.
Looking Ahead
Despite these challenges, the future of the Edge AI hardware market looks promising. With continuous advancements in hardware capabilities, such as the development of more efficient AI chips and specialized processors, the scalability and power of Edge AI devices are expected to grow. Furthermore, as cloud and edge systems continue to evolve in tandem, hybrid cloud-edge models may emerge to balance the benefits of both architectures.
The increasing demand for low-latency applications, coupled with the need for enhanced privacy, security, and energy efficiency, ensures that Edge AI hardware will remain at the forefront of the intelligent computing revolution. The key to success in this market will be addressing the challenges of cost, integration, scalability, and processing power while leveraging the vast opportunities Edge AI technology offers.
In conclusion, as the Edge AI hardware market continues to mature, businesses that can navigate these opportunities and challenges will unlock significant competitive advantages. The intersection of AI and edge computing represents a major leap forward in the evolution of intelligent devices and systems, and those who can harness its power will be well-positioned for success in the digital age.